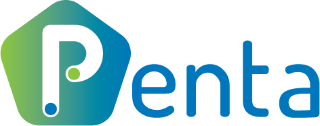
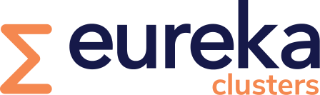
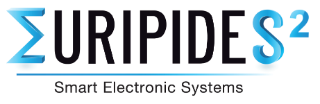
Project Abstract
Fresh water is a scarce resource essential for human survival. Globally, only 2.5% surface water is potable. This makes reliable water quality monitoring in water distribution networks very important. The major disadvantage of conventional water quality monitoring systems is that they are unable to determine and classify the type of contaminant present in the water. Therefore, novel advanced online sensor systems are needed utilizing new data analytics and classification technology.
The Aquatic Qualification with AI (or: AQUA) consortium, consisting of an experienced engineering company (NL), a data intelligence scale-up (NL), two tech-providers (NL, SG), and a large water company (BE), aims to achieve beyond-state-of-the-art real time water event detection and classification. Towards this end, advanced machine learning (ML) frameworks will be developed and integrated into two state-of-the-art sensor systems (EventLab and AquaTEC), and subsequently integrated in a single system (the AQUA-platform). The ML-frameworks will be developed to perform sensitive monitoring of physiologically relevant changes in water quality at local nodes or at the network node level, for both centralized and decentralized water production and distribution networks. The goal is to enable near-real time water quality monitoring, allowing water utility companies and industry to rapidly assess threat levels of adverse water quality events and perform the required actions for mitigation.
The annual global expenditure on drinking water production and distribution currently exceeds 180 billion USD. Within that market, the segment for water quality monitoring and analysis constitutes an estimated volume of 3.75 billion USD for 2020 and is expected to reach USD 4.68 billion by 2025. The novel ML-based sensor platforms will be exploited on the Singapore and Taiwan water quality monitoring markets in the short term and globally in the mid-to long-term.
Key facts
Start
Duration
Total budget
Partners
Countries
Coordinator: Demcon
Consortium and partners
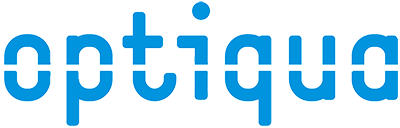
Optiqua
www.optiqua.comOptiqua (NL) provides 24/7 water quality monitoring with its EventLab system, using patented lab-on-chip sensor technology. In the project, Optiqua will enhance the EventLab Sensor Platform, integrate additional sensors, develop a machine learning framework with DEMCON, and support data collection with EventLab-ML sensors at Watergroep.
Demcon
www.demcon.comDemcon (NL), a contract R&D company with over 1100 employees, specialized in complex system design across various engineering fields. In this project, Demcon will develop a closed-circuit pipeline setup, a data platform to integrate sensor data, and collaborate with Optiqua on the ML framework and to develop trigger functions for the EventLab system that can control an autosampler to hold a sample, leveraging its expertise in sensor systems, machine learning, and system integration.
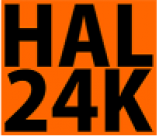
Hal24k
www.hal24k.comHal24k (NL) provides real-time insights and predictive intelligence to water utilities and businesses through advanced data science techniques and its SaaS platform, Dimension. For the project, Hal24k will supply the AI platform, advanced ML/AI models, and data science expertise, leveraging its Dimension platform to develop and operationalize data science models for business users.
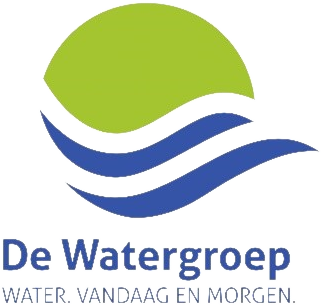
De Watergroep
www.dewatergroep.beDe Watergroep (BE), the largest drinking water company in Flanders, Belgium, supplies high-quality, affordable drinking water. In this project, De Watergroep will integrate real-time monitoring data with static model information to classify water quality sensor signals, crucial for decentralized facilities, and will provide a test environment while acting as the end customer.
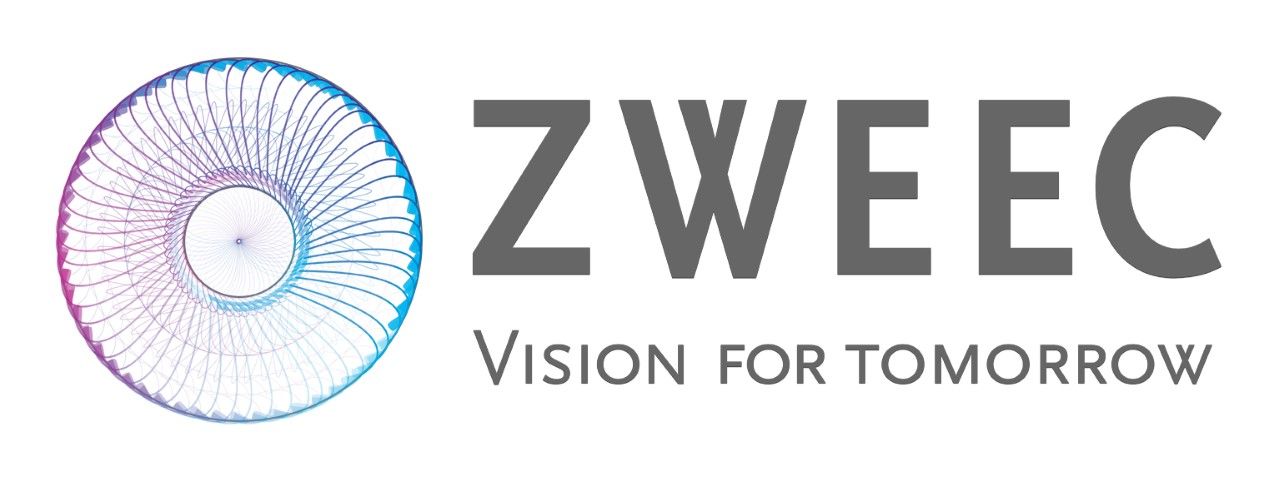
Zweec
www.zweec.comZweec (SG), a Singaporean tech company, is specialized in innovative solutions for environmental and water resource needs. In this project, Zweec will further enhance their advanced fish monitoring system AquaTEC by integrating additional water quality sensors, develop a machine learning framework, and conduct small-scale deployments in Singapore and/or Europe (BE) for data collection and validation.
The
Aqua AI
project
Our goal
The AQUA project aims to develop machine learning frameworks capable of correlating data from water quality and process monitoring sensors at both local and network levels. This innovative approach aims to move beyond the current state-of-the-art by integrating sensor information to create a digital twin of the water production and distribution network. This digital twin represents the ultimate goal in water monitoring technology, providing comprehensive insights into water parameters and facilitating proactive management of water quality and network operations.
The challenge
Ensuring the safety and reliability of water distribution networks is a critical global challenge, especially given the potential risks posed by contaminants and adverse incidents. With approximately 71% of the Earth's surface covered in water, only a mere 2.5% is fresh water, highlighting the scarcity of this essential resource. Each year, the domestic sector, industry, and agriculture consume approximately 4,000 trillion liters of potable water worldwide, emphasizing the immense scale of water usage and the importance of maintaining its quality.
Current monitoring systems often fall short in promptly detecting and accurately identifying water quality events, leaving utilities unable to respond effectively to protect consumer health. Traditional sensors lack the sensitivity and specificity needed to discern the nature and origin of contaminants. Moreover, standard data analytics struggle to interpret the complex, nonlinear datasets generated by multiple sensor systems. Without real-time actionable information, utilities face delays in implementing response protocols, jeopardizing the quality of potable water supplied to consumers and highlighting the urgent need for advanced technology solutions in water quality monitoring.
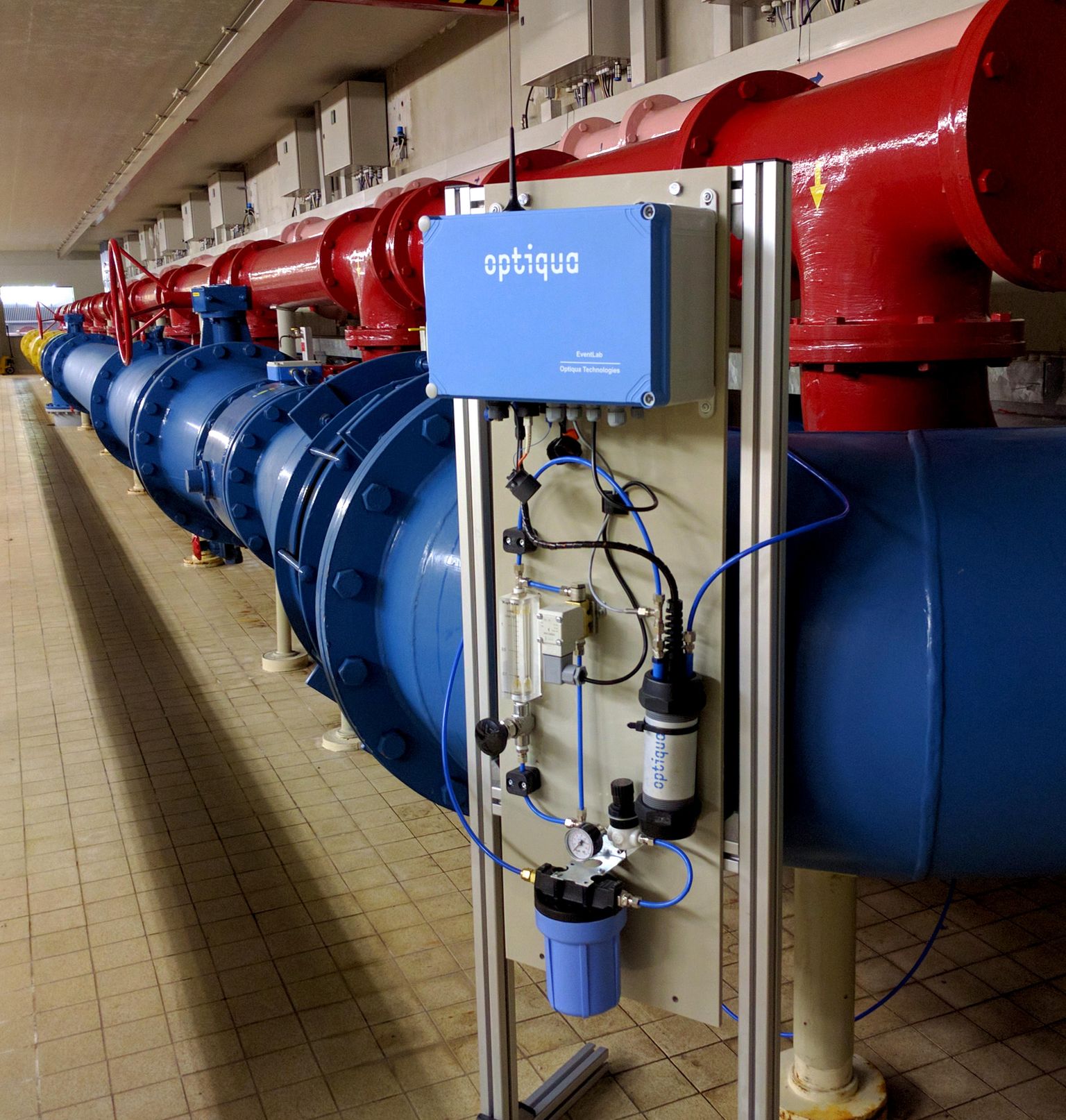
Project Innovations and Solutions
Enhanced Sensor Platforms:
Development of ML frameworks for existing sensor platforms (EventLab, AquaTEC) to perform local and sensitive water quality monitoring, enabling early detection of contaminants.
Decentralized Water Quality Monitoring:
Creation of ML frameworks to interpret real-time data from decentralized water production systems, crucial for maintaining water quality during fluctuations in water sources.
Behavior-Based Monitoring:
Implementation of ML-based machine vision frameworks to analyze fish behavior, enhancing sensitivity to physiological changes in water quality and enabling faster response rates.
Data Platform Development:
Integration of Event-Lab and AquaTEC data into a data platform. The platform will be able to collect information from additional sensors integrated with ML-frameworks for classifications of water quality.
Network-Level Data Analysis:
Development of ML-based data analysis systems for interpreting sensor data at the network level, offering insights into water quality events' origin and nature.
Water Quality Monitoring Pilot:
Conducting a pilot test in the water distribution network, validating the effectiveness of ML frameworks in detecting and classifying water quality events in real-world scenarios.